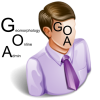
Landforms, the result of erosion and other geomorphologic processes at the surface of Earth’s crust, have a significant control on soil cover forming and evolution. In the soil-forming equation of Jenny (Jenny, 1945) the “topography” is considered a independent soil system/terrain/environment property, becoming a soil-forming factor.
The control is either direct, by influence of geomorphometric variables or geomorphologic processes on soil properties (slope on erosion/deposition and drainage), either indirect, by the influence of landforms on the other environment conditions, which also control soil cover (slope and aspect on soil temperature and humidity).
In this context, delineation of landforms is used frequently in soil mapping, using soil land systems (Huggett, 1975), were topography has a strong control on soil cover. Geomorphometric variables strongly correlate with soil property, being very used as covariate in soil properties modelling (N. McKenzie, 1999; N. J. McKenzie, Gessler, Ryan, & O'Connell, 2000; Tanasa, Niculita, Rosca, & Pirnau, 2010).
We present here the results of several geomorphometric techniques which can be used to enhance the usability of landform indicators from Romanian Soil Study Methodology (Florea et. al., 1987a, 1987b, 1987c)
In Romanian soil study methodology (Florea, Bălăceanu, Răuță, Canarache, & (coords.), 1987a, 1987b, 1987c), landforms type are used for characterizing pedologically the terrain. For this purpose a set of indicators are used to classify the terrain in several classes. Landforms are used at macro-, meso- and micro-scale, for pedologic micro-zones characterization (indicator 1, 2, 6, 8), for delineating soil mapping units (indicator 20) and for delineating terrain mapping units (indicator 31, 32, 33, 34, 37, 38, 40).
For indicator 1, the pedologic micro-zones are delineated using some generalized types of landforms together with climatic zone and major soil. These generalized qualitative landforms are:
- floodplains;
- smooth (tabular) lowland;
- rolling relief;
- low roughness;
- mean roughness;
- high roughness.
Indicator 2 classify principal landforms as:
- mountains;
- hills, table lands and fragmented piedmonts;
- plains (including terraces), table lands and non- or weak fragmented piedmonts;
- terraces not in plain areas;
- floodplains, divagation plains, deltas, coastal plains.
For the delineation of generalized landforms of pedologic micro-zones from indicator 1 and of generalized small scale landforms from indicator 2, several approaches can be used. Floodplain can be delineated using altitude above channel network/available relief (Dury, 1951)/drainage relief (Glock, 1932), as the area which is vertically very close to channels. Roughness of the terrain surface can be assessed using vector ruggedness measure (Sappington, Longshore, & Thompson, 2007). Qualitative small scale classifications (Hammond, 1954, 1964; Iwahashi & Pike, 2007) can be used in this approach to obtain a complete series of general landform classes.
We propose the following data for indicator 1
We propose the following data for indicator 2
For indicator 6, the absolute altitude is classified in 100 m intervals from 0 to 1200 m, while for 1200 to 2000 m in 200 m intervals and the last class cumulate altitudes bigger than 2000 m.
An analysis of altitude distribution using SRTM3 data for entire Romania can tune the classes of absolute altitude from indicator 6 by applying Jenks algorithm (Jenks, 1967).
For indicator 9 the local micro-relief (altitude amplitude) which needs to be leveled is used to derive 6 classes of non-uniformity.
The local micro-relief altitude amplitude from indicator 9 needed to obtain non-uniformity classes can be modeled only using high resolution elevation data, like LIDAR, GPS or stereographic restitution. Image analysis of aerial ortorectified imagery can also be used to substitute altitude data, using the shading introduced by the non-uniform large scale topography.
Indicator 31 classify the shape elements of principal landform as:
- horizontal smooth areas (slope
- horizontal rough areas (slope 20 cm and quasi-horizontal (slope 1-2 %) with relief 20 cm;
- slightly sloped (slope 2-5%);
- ridge: very large (width bigger than 100 m - plateaus), large (width 30-100 m), narrow (under 30 m width) and crests;
- smooth hillslope: long (length > 200 m) and short (length
- rough hillslope: long, short, terraced;
- steep slope;
- floodplain: high floodplain, transitional floodplain and low floodplain.
For the surface shape elements classification of indicator 31 a mixed approach of (Schmidt & Hewitt, 2004) 15 classes classification, catena based classifications and geomorphometric variables threshold can be used.
Indicator 32 classify the meso- and microlandforms as:
- peak;
- saddle;
- aeolian landform: dune and interdune;
- mound;
- erosion remnants;
- glacial or nival cirque;
- karst landforms: lapiez, sinkholes, poljes;
- depressions: micro-depressions, closed loess depressions, inter-micro-depressions, large depressions
- fluvial morphology: alluvial cones, debris cones, glacis, natural levees, back-swamps, paleochannels, cut-offs, river channel, drainage channel, ephemeral channels, gullies, rills;
- badlands;
- landslide micro-landforms;
- gilgai micro-landforms;
- animal path;
- shape of the hillslope: upper hillslope (shoulder), median hillslope (backslope), toe slope, hollow, secondary rigdes on hillslopes;
- anthropogenic landforms.
The meso- and micro-landforms from indicator 32 can be delineated using geomorphometric techniques, but some landforms require extra information like geologic maps, aerial ortorectified images or other sources.
Peak and saddles can be identified using several neighborhood or multiscale approaches (Peucker & Douglas, 1975; Wang, Laffan, Liu, & Wu, 2010; Wood, 1996).
Shape of hillslope can be assessed using Pennock-Reuter classification (Reuter et al., 2006). Large scale depressions/hollows can be identified using a D8 flow algorithm, but there are serious problems, because in DEMs depression are considered artifacts, additional data being necessary to validate the depression presence. Only high resolution DEMs and karst areas can contain pure geomorphometric large scale depressions. On the other hand small scale depressions can be easily identified using geomorphometric criteria (Shary, Sharaya, & Mitusov, 2002).
Indicator 33 classify slope (in %) in the following categories: ≤ 2, 2-5, 5-10, 10-15, 15-20, 20-25, 25-35, 35-50, 50-100, > 100.
The 10 slope classes from indicator 33 can be somehow arbitrary as class limits, although in a previous study we have showed that for some areas in Iasi county, a histogram analysis of slope showed 9-10 classes, but with different thresholds (I. C. Niculita & Niculita, 2011). Jenks algorithm for example (Jenks, 1967) can be used to find the thresholds for a given areas considering 10 as the optimum number of classes.
Indicator 34 classify aspect (exposition) in the following categories: flat, N and NE, E and NW, S and SW, W and SE, all aspects.
Aspect classes from indicator 34 are based on climatic considerations, the single problem on computing this variable from DEMs, being the presence of flat areas in which the aspect cannot be computed. Real flat areas, especially in small scale DEMs like SRTM can be considered artifacts. Contour interpolated DEMs can present a large proportion of flat areas artifacts.
Indicator 37 classify rill erosion categories based on the depth of the rill or gully.
Indicator 38 classify the categories of landslides using the morphologic approach (the shape of the landslide body) and the actual rate of the process (stabilized, semi-stabilized, active).
Indicator 40 classify the categories of flooding activity using the temporal approach (yearly, 2 to 5 years and more than 5 years flooding frequency).
References
A-Xing, Z. H. U., Burt, J. E., Smith, M., & Rongxun, W. (2008). The Impact of Neighbourhood Size on Terrain Derivatives and Digital Soil Mapping. In Q. Zhou, B. Lees & G.-a. Tang (Eds.), Advances in digital terrain analysis: Springer.
Becek, K. (2008). Investigating error structure of shuttle radar topography mission elevation data product. Geophysical Research Letters, 35(15), 1-5.
Bonin, O., & Rousseaux, F. (2005). Digital Terrain Model Computation from Contour Lines: How to Derive Quality Information from Artifact Analysis. GeoInformatica, 9(3), 253-268.
Dehn, M. (2001). Principles of semantic modeling of landform structures. Computers & Geosciences, 27(8), 1005-1010.
Dragut, L., & Blaschke, T. (2006). Automated classification of landform elements using object-based image analysis. Geomorphology, 81(3-4), 330-344.
Dury, G. H. (1951). Quantitative Measurement of Available Relief and of Depth of Dissection. Geological Magazine, 88, 339-343.
Farr, T. G., Rosen, P. A., Caro, E., Crippen, R., Duren, R., Hensley, S., . . . Alsdorf, D. (2007). The shuttle radar topography mission. Reviews of Geophysics, 45(RG2004).
Florea, N., Bălăceanu, V., Răuță, C., Canarache, A., & (coords.). (1987a). Metodology for pedologic studies elaboration. Part I - Colecting and resuming pedologic data (Vol. I). Bucharest: The Center for Educational Material and Agricultural Propaganada.(in romanian)
Florea, N., Bălăceanu, V., Răuță, C., Canarache, A., & (coords.). (1987b). Metodology for pedologic studies elaboration. Part II - Eco-pedologic indicators (Vol. II). Bucharest: The Center for Educational Material and Agricultural Propaganada. (in romanian)
Florea, N., Bălăceanu, V., Răuță, C., Canarache, A., & (coords.). (1987c). Metodology for pedologic studies elaboration. Part III - Elaboration of pedologic studies for various purposes (Vol. III). Bucharest: The Center for Educational Material and Agricultural Propaganada. (in romanian)
Glock, W. (1932). Available Relief as a Factor of Control in the Profile of a Land Form. The Journal of Geology, 40(1), 74-83.
Griffiths, J. S., Smith, M. J., & Paron, P. (2011). Introduction to Applied Geomorphological Mapping Geomorphological mapping. Methods and applications (Vol. 15, pp. 3-11): Elsevier.
Hammond, E. H. (1954). Small-Scale Continental Landform Maps. Annals of the Association of American Geographers, 44(1), 33.
Hammond, E. H. (1964). Analysis of properties in land form geography: an application to broad-scale land form mapping.
Hengl, T., & Reuter, H. I. (2011). How accurate and usable is GDEM? A statistical assessment of GDEM using LiDAR data. Paper presented at the Geomorphometry 20118 Sept. 2011, Redlands, USA.
Huggett, R. J. (1975). Soil landscape systems: a model of soil genesis. Geoderma, 13, 1-22.
Iwahashi, J., & Pike, R. J. (2007). Automated classifications of topography from DEMs by an unsupervised nested-means algorithm and a three-part geometric signature. Geomorphology, 86(3-4), 409-440.
Jenks, G. F. ( 1967). The Data Model Concept in Statistical Mapping International Yearbook of Cartography, 7, 186–190.
Jenny, H. (1945). Factors of Soil Formation: A System of Quantitative Pedology. New York: Dover Publications.
Lindsay, J., & Creed, I. (2006). Distinguishing actual and artefact depressions in digital elevation data. Computers & Geosciences, 32(8), 1192-1204.
MacMillan, R. A., Martin, T., Earle, T., & McNabb, D. (2003). Automated analysis and classification of landforms using high-resolution digital elevation data: applications and issues. Canadian Journal of Remote Sensing, 29(5), 592-606.
MacMillan, R. A., & Shary, P. A. (2009). Landforms and landform elements in geomorphometry Geomorphometry: concepts, software, applications (Vol. 33, pp. 227-254).
Mark, D. M., Smith, B., & Tversky, B. (1999). Ontology and Geographic Objects : An Empirical Study of Cognitive Categorization. Paper presented at the Spatial Information Theory. Cognitive and Computational Foundations of Geographic Information Science. International Conference COSIT'99 Stade, Germany, August 25-29, 1999 Proceedings.
McKenzie, N. (1999). Spatial prediction of soil properties using environmental correlation. Geoderma, 89(1-2), 67-94.
McKenzie, N. J., Gessler, P. E., Ryan, P. J., & O'Connell, D. A. (2000). The role of terrain analysis in soil mapping. Terrain analysis: principles and applications (pp. 245-265).
Nelson, A., Reuter, H. I., & Gessler, P. (2009). DEM production methods and sources. Geomorphometry: concepts, software, applications (Vol. 33, pp. 65-85).
Niculita, I. C., & Niculita, M. (2011). Methods for natural land mapping units delineation for agricultural land evaluation. Scientific Papers USAMV Iasi. Agronomy series, 54(1), 44-49.
Niculita, M. (2011). Effects of geomorphometric variables variability due to different computing algorithms in environmental modeling. Procedia Environmental Sciences, 7, 335-340.
Niculiță, M. (2011). A classification schema for structural landforms of the Moldavian platform (Romania). Paper presented at the Geomorphometry 2011, 8 Sept. 2011, Redlands, USA.
Niculita, M., & Rusu, C. (2010). The soil and geomorphologic limits between Copou hillslope and cacaina floodplain (Iasi Area). Soil Forming Factors and Processes from Temperate Zone, 9, 31-41.
Pennock, D., Zebarth, B., & Dejong, E. (1987). Landform classification and soil distribution in hummocky terrain, Saskatchewan, Canada. Geoderma, 40(3-4), 297-315.
Peucker, T., & Douglas, D. (1975). Detection of Surface-Specific Points by Local Parallel Processing of Discrete Terrain Elevation Data. Computer Graphics and Image Processing, 4(4), 375-387.
Reuter, H., Wendroth, O., & Kersebaum, K. (2006). Optimisation of relief classification for different levels of generalisation. Geomorphology, 77(1-2), 79-89.
Romstad, B. a. r. (2001). Improving Relief Classification with Contextual Merging. Paper presented at the ScanGIS'2001 The 8th Scandinavian Research Conference on Geographical Information Science.
Sappington, J. M., Longshore, K. M., & Thompson, D. B. (2007). Quantifying Landscape Ruggedness for Animal Habitat Analysis: A Case Study Using Bighorn Sheep in the Mojave Desert. Journal of Wildlife Management, 71(5), 1419-1426.
Schmidt, J., & Hewitt, A. (2004). Fuzzy land element classification from DTMs based on geometry and terrain position. Geoderma, 121(3-4), 243-256.
Seijmonsbergen, A. C., Hengl, T., & Anders, N. S. (2011). Semi-Automated Identification and Extraction of Geomorphological Features Using Digital Elevation Data. In M. J. Smith, P. Paron & J. S. Griffiths (Eds.), Geomorphological mapping. Methods and applications (Vol. 15): Elsevier.
Shary, P. A., Sharaya, L. S., & Mitusov, A. V. (2002). Fundamental quantitative methods of land surface analysis. Geoderma(1), 1-19.
Smith, B., & Mark, D. M. (2001). Geographical categories: an ontological investigation. International Journal of Geographical Information Science, 15(7), 591-612.
Smith, B., & Mark, D. M. (2003). Do mountains exist? Towards an ontology of landforms. Environment and Planning B: Planning and Design, 30(3), 411-427.
Tanasa, I. C., Niculita, M., Rosca, B., & Pirnau, R. (2010). Pedometric Techniques in Spatialisation of Soil Properties for Agricultural Land Evaluation. Bulletin UASVM Agriculture, 67(1), 274-278.
Wang, D., Laffan, S., Liu, Y., & Wu, L. (2010). Morphometric characterisation of landform from DEMs. International Journal of Geographical Information Science, 24(2), 305-326.
Wise, S. (2000). Assessing the quality for hydrological applications of digital elevation models derived from contours. Hydrological Processes, 14, 1909-1929.
Wood, J. (1996). The geomorphological characterisation of digital elevation models. University of Leicester.
Zhou, Q., & Liu, X. (2004). Error analysis on grid-based slope and aspect algorithms. Photogrammetric Engineering & Remote Sensing, 70(8), 957-962.
- Log in to post comments
Recent comments